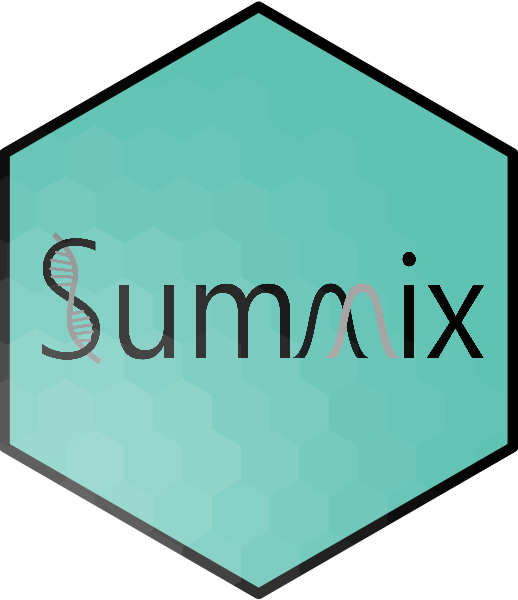
Summix2 is a comprehensive set of methods and software based on a computationally efficient mixture model to estimate and adjust for substructure in genetic summary data. In extensive simulations and application to public data, Summix2 characterizes finer-scale population structure, identifies ascertainment bias, and identifies potential regions of selection due to local substructure deviation. Summix2 increases the robust use of diverse publicly available summary data resulting in improved and more accurate research for all people and populations.